Me AI, you Jane!
DigitizationThe MUHAI research project aims to make AI understandable to human beings
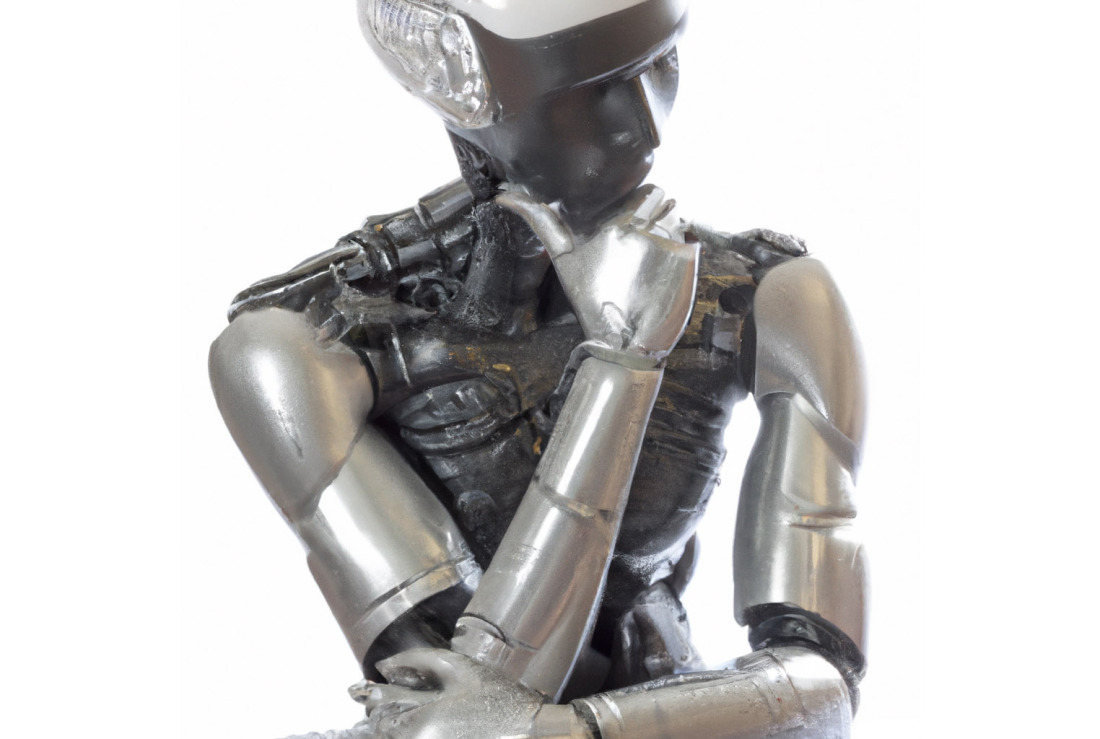
Whether it's homework tasks, programming code or as a relationship advisor, there's no area of life that ChatGPT is unfamiliar with. In recent weeks, this AI system has been widely discussed in the media and is already used by many people in everyday situations - according to a recent study, 100 million users interacted with the AI in January 2023.
But it has its flaws. It is confidently incorrect – it confidently gives even completely wrong answers that often can only be classified as nonsensical by experts. It doesn't understand what it's talking about, it just gathers content from the internet in a highly complex way. Mostly pretty well, but only mostly.
Public appearance by Rainer Malaka and Robert Porzel. These two scientists from the University of Bremen are part of the MUHAI – "Meaning and Understanding in Human-centric AI" project. Behind the project title is a new application for artificial intelligence: "We want to give AI a human viewpoint, and the key to this is understanding language", stated Prof. Rainer Malaka, Managing Director of the Technologiezentrum Informatik und Informationstechnik (TZI: the Centre for Computing Technologies).
The parrot that doesn't understand anything
To understand what this means, we need to go into a bit of detail. AI systems such as ChatGPT are a type of machine learning system. This is a system that can learn, completely autonomously, to recognise a pattern from an existing data set and then apply this pattern to new situations. The more data these systems have, the better they will become. ChatGPT uses many billions of texts taken from the Internet to create its responses. These responses are new. The program doesn't simply copy texts from somewhere or other, although its responses are inevitably based on existing data.
"However, an AI system like this can't explain why it gives a particular response, even if it's the correct one", said Malaka. This is because the response is rooted in its programming, in the interplay of thousands of lines of code, which have been trained to identify specific patterns and then to reproduce them.
"Basically, the AI system is a statistical parrot", he said, giving this example: "I used to know a parrot which would shout: 'I'll be right there!' every time the doorbell rang because that's what its owner often said in this situation. Although this response was factually correct, the parrot didn't know why it said it. And it said the same thing even when no-one else was at home."
Just like the parrot, AI systems like ChatGPT can babble the correct responses without knowing the meaning behind the words.
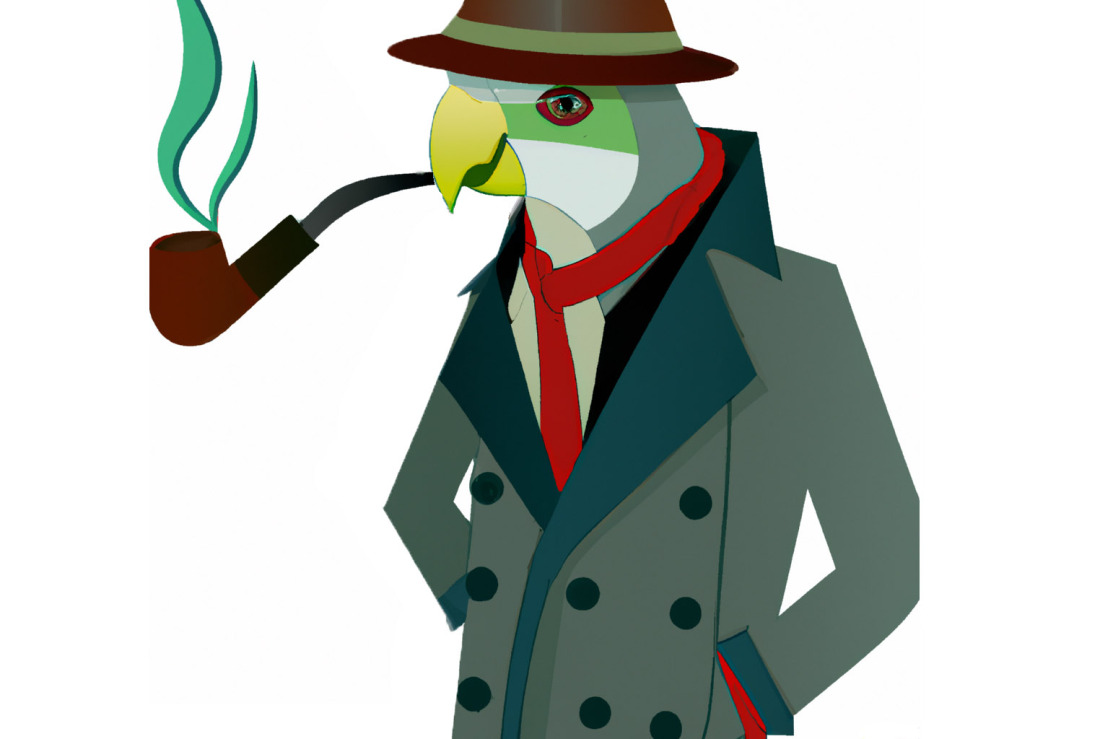
A parrot with deerstalker, cloak and pipe
And this is exactly where MUHAI comes in. Among other things, this eight million Euro project is investigating an approach which has recently fallen out of favour: symbolic AI. This type of AI doesn't learn to recognise a pattern independently, from its training data (a process also known as sub-symbolic learning). Instead, it uses logical conclusions based on a specific set of data. It's like Sherlock Holmes in that it draws the correct conclusions from a set of known facts.
However, MUHAI goes even further. This AI system isn't just able to set facts in context: it can use them to generate a simulation of real events which it then uses to draw conclusions about its environment.
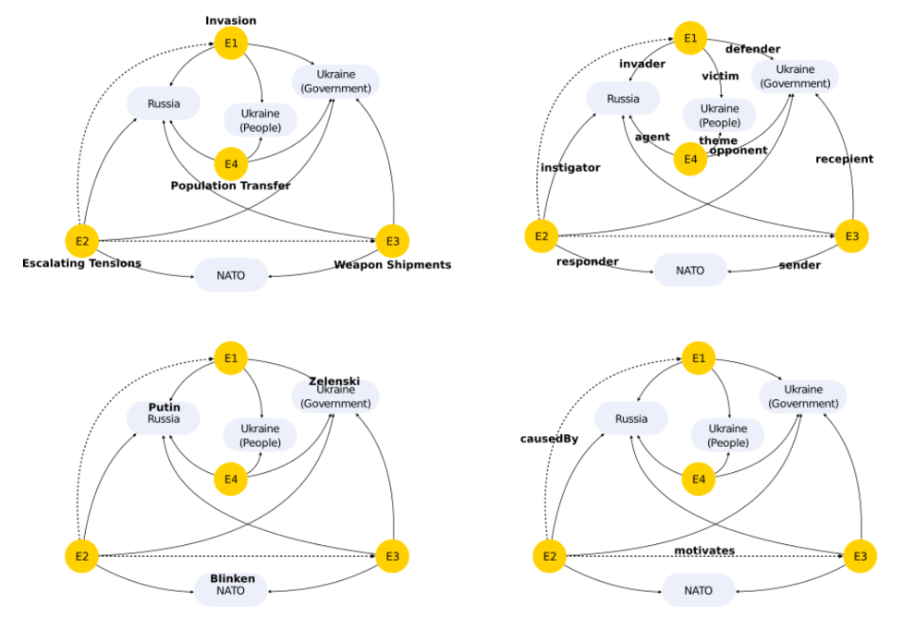
What can an AI system cook up? Alphabet soup!
Cooking recipes are a good way of illustrating exactly what this means and they are one of the two areas used by the MUHAI European research project as a means of testing the AI system's capabilities.
"Recipes are a real challenge for an AI system", said Dr. Robert Porzel, research associate of the Digitale Medien working group and member of the MUHAI project at the University of Bremen. "That's because recipes are based on common knowledge and an understanding of physics which are not actually described in the text itself."
For example, a recipe might say:
- Mix together butter, sugar, milk, eggs and flour.
- Pour the batter into a loaf tin.
- Bake for 20 minutes at 175 degrees.
A human being would understand this immediately, whereas an AI would simply give up. Where does this batter come from? It's not in the list of ingredients. "It's also not clear who is actually baking what and where. All of this knowledge is taken for granted by the recipe's creator", said Porzel.
The MUHAI AI system is able to understand these instructions because it inserts each step into a complex language model which can determine the relationships between the individual words. It then enters the results in a computer simulation which reproduces the recipe in a type of computer game.
"By actually using the recipe to cook something in a realistic environment, the computer is able to understand what is actually going on. If the recipe says "cover the pan to prevent heat escaping", but there isn't a pan lid nearby, the AI system could use a plate or a spatula. However, in the simulation, the computer would notice that heat would still escape if a spatula were used and that only a plate would be suitable. This means the AI system will have discovered something new, which it could then apply directly next time", continued Porzel. As a result, this AI system is more robust than a purely sub-symbolic AI system, which either gives up or produces nonsense when it encounters situations which don't fit in with its training data.
Obviously, this combination of a language model and a simulation requires the appropriate databases, which contain both terms and relationships. MUHAI uses a database from a supplier in Amsterdam, which holds ten billion facts and the relationships between them. The system can also be combined with sub-symbolic AI systems, for example neuronal networks such as ChatGPT, which gives it an even greater pool of data.
A new step for AI
This approach will also make AI easier to understand for human beings. Porzel: "I can ask the AI system: Why did you use the plate? And the AI system replies: To stop the steam from escaping. This is a great step forward." And that is also MUHAI's goal: to create an AI system that people can understand.
The team of scientists believe this will make artificial intelligence more approachable. After all, using language to make sense of our surroundings is a central function of our consciousness. We know what words mean, but computers don't. MUHAI wants to tear down this barrier. "We're taking the first steps in an entirely new field, by creating a new way of thinking for an AI system. That's a task which appeared to be insoluble for so long", said Porzel.
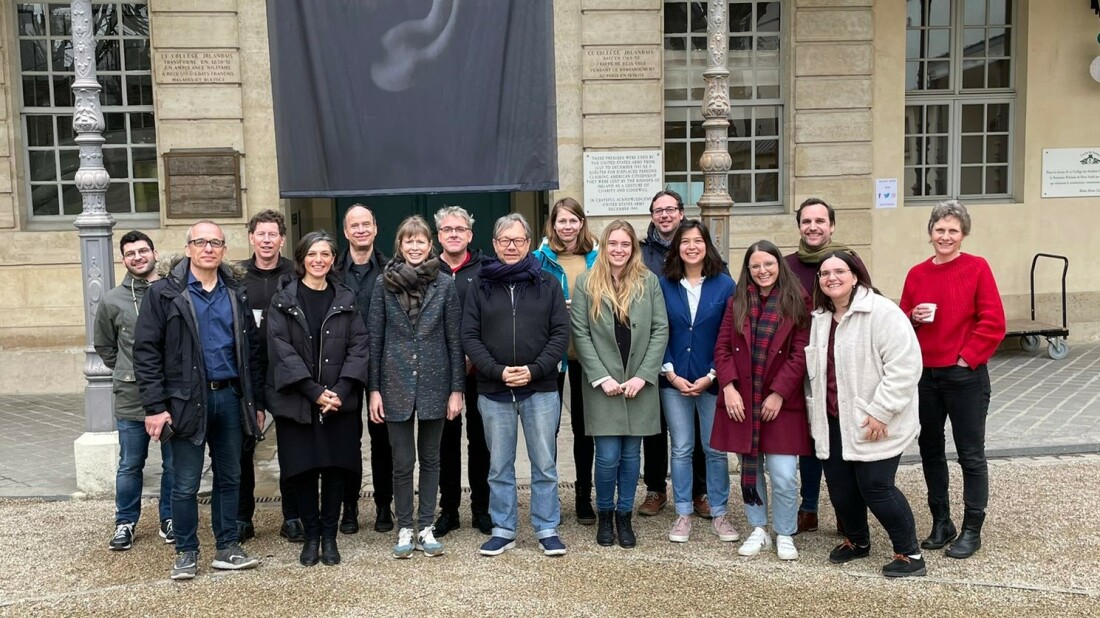
Use in real kitchens
So how could this be useful? The team of 40 scientists in Bremen, Venice, Amsterdam, Brussels, Paris and Barcelona aren't just teaching an AI system how to cook to satisfy their curiosity. apicbase, a company based in Belgium, is also taking part in this research project. It wants to use AI to reduce food waste. "This software could be used in restaurants, where it would learn recipes and work together with the food purchasing systems to calculate the quantities of ingredients required or suggest recipes for dishes that could be made from left-overs. Restaurants then wouldn't have to throw so much food away", said Porzel.
Battling against disinformation and fake news
Another area in which MUHAI could be used are social media networks, in particular, Twitter. "Conventional AI systems find tweets very hard to analyse because they are short messages that each reference one another without alluding to their content specifically. They use a very abbreviated form of language and there is a constant and dynamic flow of content which the AI systems have not been trained to recognise", stated Malaka.
Sub-symbolic AI systems are poorly equipped to react to the completely new, rapidly changing sets of contents or handle one-off questions which have no previous examples or similarities to earlier events.
This is where the MUHAI language model comes into its own by dissecting and analysing the statements made on Twitter and putting the component parts into context with each other. By analysing thousands or even millions of Tweets, the AI system is able to recognise streams and patterns that are far too complex for a human being to identify. "This enables us to uncover targeted disinformation campaigns or networks in which radical content is being shared. It will also help us understand how and where influencing takes place", said Malaka, looking to the future.
Before the project comes to an end in 2024, the research team aims to publish a range of tools, software components and guides which can be used and further developed by journalists, free of charge.
"We're not aiming to replace sub-symbolic AI but instead create an add-on that can solve problems which are currently insoluble. We have discovered an approach which combines symbolic and sub-symbolic procedures to create architectures that can simulate the depth of understanding of human language. Others can then build upon our findings", said Porzel.
Bremen: the ideal starting point for European cooperative ventures
MUHAI has received funding from Horizon 2020, the European Union research programme. Coordinated from Bremen, the project's partners include the Venice International University, the Vrije Universiteit (VU) Amsterdam, the Free University of Brussels, the Sony Computer Science Laboratory Paris and the company apicbase.
According to Malaka, the project fits in perfectly with the core areas of AI research in Bremen. "When creating the simulation, we drew heavily on Bremen's key AI expertise in robotics and also on the media research and communication sciences represented here at the university. The project is also very much at home as part of Bremen's AI strategy, which deliberately places human AI firmly in the foreground. So, here we have an AI system which uses human beings instead of regarding them merely as data points."
MUHAI's AI system doesn't need quantities of data harvested from Facebook or Amazon etc. because it can make decisions based on the facts supplied by its real environment, by processing them and understanding what it experiences.
Malaka can also imagine the system having closer links with industry in future. There would be the initial contact with the Bremen AI Transfer Centre which has set itself the task of facilitating the transfer of knowledge between the research community and industry. The food and beverage industry in particular could benefit from this new type of AI, said Malaka. And to conclude: "This project is a mark of Bremen's excellence and proof that innovative approaches can lead to success."
Success Stories
“After all, we're here because someone else made room for us, and it's our duty to do the same for others”
Theoretical physicist, industrial mathematician, manager – as a member of the start-up company TOPAS, Dr. Shruti Patel creates change in Bremen. However, being a role model has not always been easy for her.
Learn more12 Examples of AI in Medicine and the Health Sector
Detecting cancer earlier or avoiding accidents at work - AI will make our lives easier and easier in the healthcare sector. In Bremen, a strong AI landscape is developing in the healthcare sector, as these 12 examples show.
Learn moreThese Bremen companies are developing autonomous systems
Self-driving cars, drones, robots - in Bremen, autonomous systems are a focus of numerous focus of numerous companies. A look at the diverse corporate landscape and where the committed players can be found.
Learn more