The "thinking" 3D printer: machine learning combined with 3D printing for monitoring the quality of components
ECOMAT / lightweight construction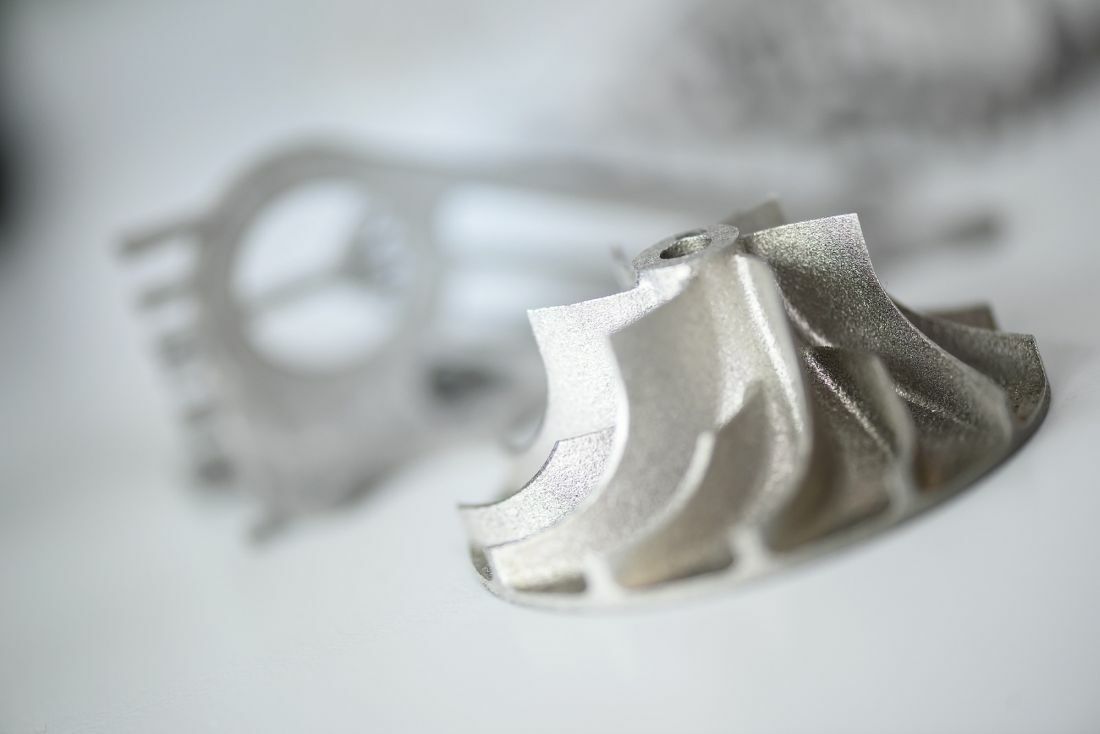
In sectors where the safety requirements are exceptionally high, such as aviation, errors in manufacturing components must be prevented at all costs. The cost involved in checking components for flaws is always a significant factor. A project based in Bremen is creating an algorithm that will work as an adjunct to visual inspections in this sector.
In recent years, 3D printing has moved on from prototyping to being used in industrial applications, even in sectors with exceptionally high safety requirements, such as aviation and aerospace. Nowadays, a number of extremely complex components are produced using additive manufacturing, often from materials such as titanium.
These printed components are not always perfect: they can have cracks, pores or inclusions of other materials which could cause critical failures. For this reason, additively manufactured components undergo a thorough inspection process (as do components produced using "traditional" methods) before being installed in an air plane.
These inspections are performed by specially trained and certified inspection engineers, who use methods such as X-ray computed tomography to screen components.
Joint research project run by ECOMAT members
"Inspection costs are enormously high and can account for up to 50 percent of a component's total costs", said Stefan Neumann, Data Scientist at Testia GmbH, a Bremen company specialising in material inspection, training courses for inspection personnel and engineering, primarily in the aviation sector.
Neumann is part of the "Automated fault detection and evaluation using X-ray computed tomography (CT) data for highly complex 3D metal and fibre composite components" research project, which Testia is running together with the Faserinstitut Bremen (Fibre Institute, Bremen) (German) and the company Kolbes Messtechnik (German) up to the end of 2021.
The objective of this research project is to reduce the enormous amount of time and money involved in inspections and to make additive manufacturing affordable for applications in which its use was previously too expensive.
How algorithms learn to identify flaws
In their efforts to automate the identification of flaws and errors in future, the research team has combined two approaches: one is computed tomography (CT), which is used after a component has been manufactured. It shows where faults have occurred in the component. "International regulations require us to be able to detect very small faults, only slightly thicker than a human hair", said Neumann.
To do this, the CT data is correlated with data gathered by sensors during the printing process itself. "The laser's position is recorded, as is data from other sensors which measure, for example, sounds, temperature and radiation", he went on to explain.
The CT data and sensor data are transferred into a neural network. "Our goal is to teach this neural network how to use the sensor data collected during the 3D printing process to predict the position and size of faults."
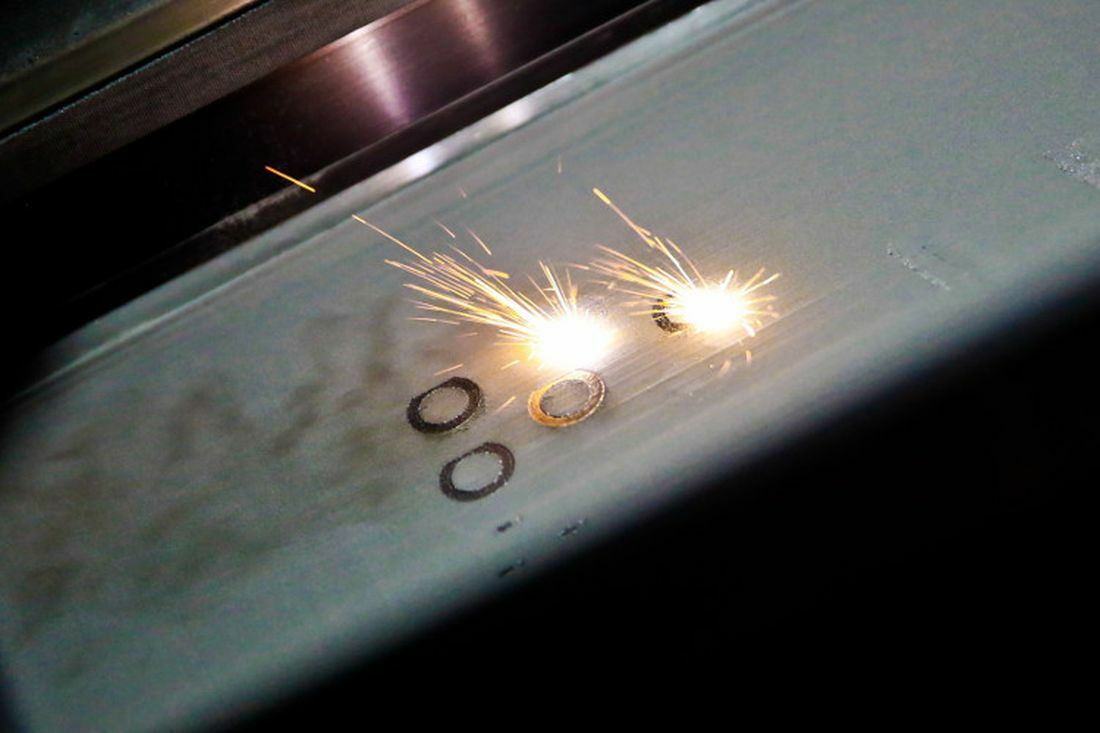
Implementing machine learning reliably, even in safety-critical applications
In future, faults could be detected more quickly, or even prevented entirely, by having the neural network automatically issue an alarm during the 3D printing process, and stop it, as soon as a fault source is identified.
One day, a machine learning system could even be able to perform material inspections on its own, without any human help. However, that day is still some distance away for the research team in Bremen. "We want to help inspectors detect flaws more quickly and more reliably", said Neumann.
The project group still sees itself as facing a major challenge: How can working with machine learning achieve an acceptable success rate for being used in the aviation industry? In other words, how can it be ensured that it actually finds every fault and doesn't miss anything? "This is a general challenge faced by AI in every application, whether it is self-driving cars or in the aviation industry, which obviously has even more exacting requirements than in other sectors."
The problem is not only technically challenging, but also needs adjustments to be made to regulations, by bodies such as the European Union Aviation Safety Agency (EASA).
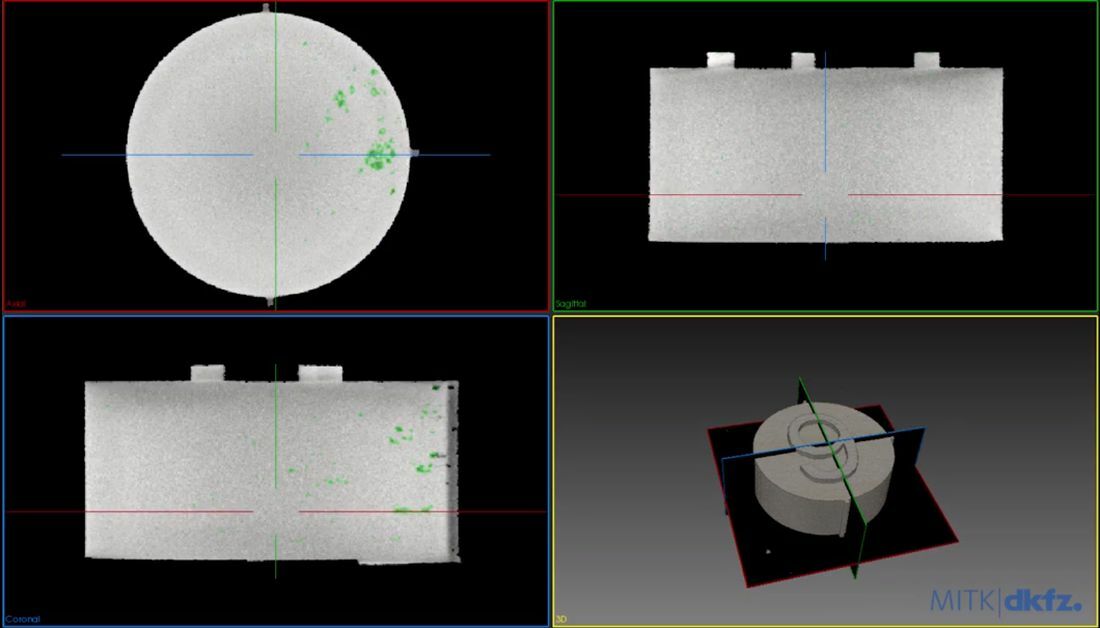
Working together in Bremen to advance 3D printing technologies
However, this is no longer part of the Bremen-based research project, which concentrates exclusively on investigating options for automating the detection of faults. When the project finishes, at the end of 2021, it will demonstrate that, in principle, machine learning can be implemented in 3D printing. The project was set up and run as part of the Luft- und Raumfahrtförderprogramm Bremen (Bremen aviation and aerospace funding program) supported by the BAB (Bremen's development bank). It was also supported by the European Regional Development Fund (ERDF). This project shows just how well companies, institutes and other bodies in Bremen can work together in the field of material sciences.
A secondary benefit: a new business model
This process is an important step into the future of materials inspection for the Testia, which is a subsidiary of Airbus. In addition to developmental research, the project has a secondary benefit for the company:
"The developments brought about by working on the project have led to the creation of new computed tomography software. This new programme uses the individual cross-section images taken by the computed tomography process to generate a three-dimensional image. This can then be used to display faults or show the interior of a component", explained Neumann, who is both a physicist and an engineer.
Similar technology is already being used in industry and medical applications, but the most commonly used software in these sectors is currently extremely expensive. "We've already had a number of enquiries about our newly developed software", he explained. The first fully functional prototype will be ready at the end of 2021, and will open up a whole new line of business for Testia.
Success Stories
Take-off for Bremen: what makes the city a hub for aerospace expertise
The sky is not the limit. Science, production, development and business all agree on this. Aircraft wings, Ariane rockets, Galileo satellites - Bremen is one of the top locations in the international aerospace industry. Five reasons for Bremen's success story.
Learn moreFrom the sun of southern France into the northern German summer: an internship at ECOMAT
What is it like to be a intern at the technology centre ECOMAT? Éva Perrier comes from Montpellier – and quickly finds her feet in Bremen. What she loves about Bremen.
Read more at ECOMAT“As a small company, being a member of ECOMAT opens new doors for us”
Gaining a foothold in new sectors and new target groups is often difficult for small companies. Michel Petkovic was lucky enough to achieve this step with his start-up WeserCAD. To achieve this, the company that specializes in 3D printing has become a member of the ECOMAT.
Learn more